Bridging technology and healthcare, the SCENARIOS project, funded by the EU’s Horizon 2020, pioneers advancements in risk assessment and environmental health through synergistically blending computational chemistry, cheminformatics and machine learning, with deep learning. It introduces innovative tools for predicting molecule-biological target interactions and compound cytotoxicity, aligning with the European Green Deal’s toxin-free vision. These models combine computational power with user-centric designs, enhancing global research capabilities.
All models are hosted on the Enalos Cloud Platform (Figure 1), featuring user-friendly interfaces that allow researchers to input chemical structures in various formats and receive predictions instantaneously. The platform is free to use and there is no need for authentication to access the tools. Training material is also available to help the users to exploit the models. This accessibility underscores the project’s commitment to democratizing advanced computational tools for the scientific community.
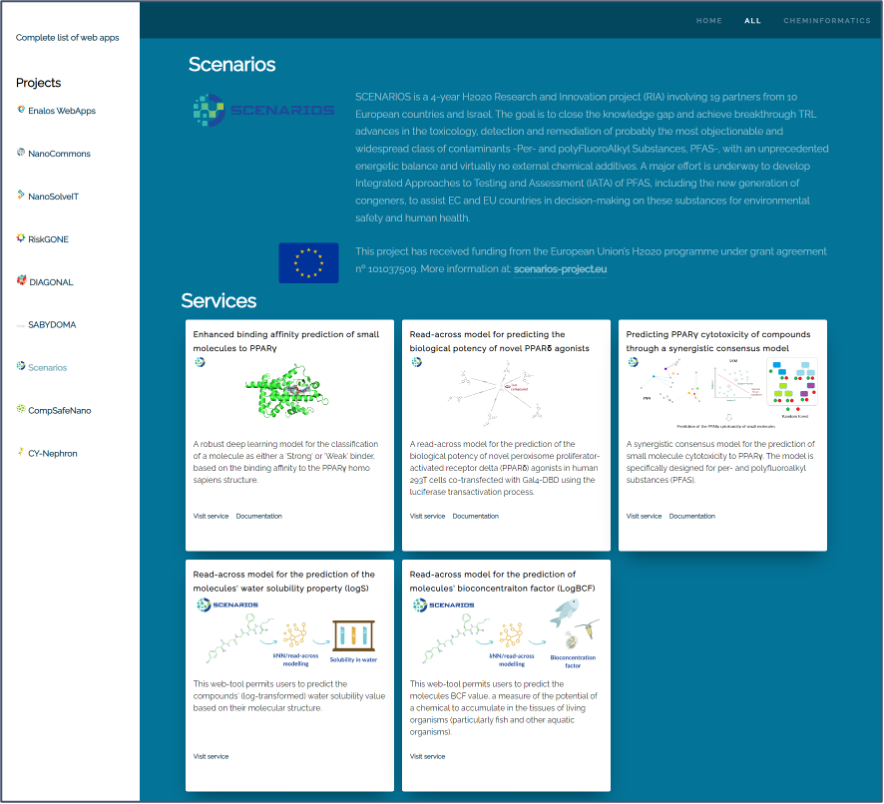
As we investigate deeper into the specifics of the SCENARIOS project, it is essential to recognize its role not just in assessing long-term exposure risks of Per- and Polyfluoroalkyl Substances (PFAS) but also in setting new standards for environmental stewardship and health protection. Through the fusion of deep learning and scientific inquiry, the project paves the way for a future where technology and healthcare converge to overcome the challenges of the present and unlock the possibilities of tomorrow. Some of the key developments made up to now during the SCENARIOS project include:
Biological Potency Prediction for PPARδ Agonists: Adhering to OECD guidelines, this read-across model utilizes PubChem bioassay data to predict the biological potency of novel PPARδ agonists, aiding in the identification of compounds with therapeutic potential (Link to service, Link to Documentation).
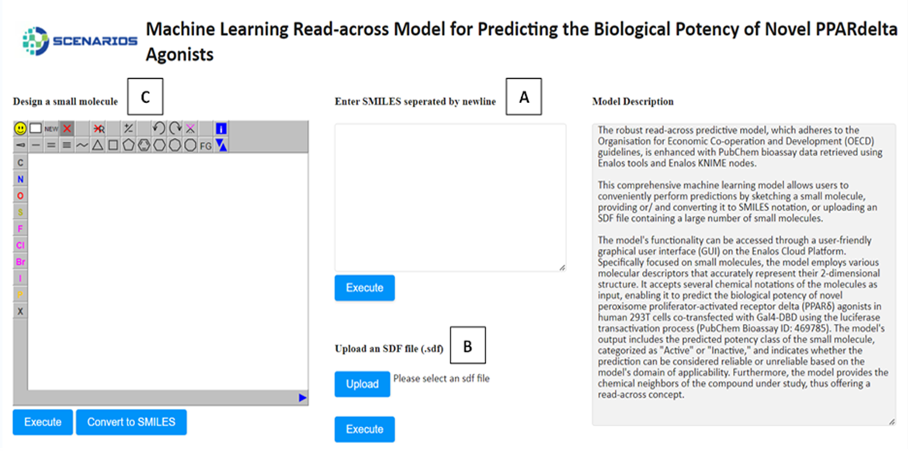
Enhanced Binding Affinity Prediction small molecules to PPARγ: Leveraging the power of the DeepChem package and Keras DL framework, this model predicts the binding affinity of small molecules to Peroxisome Proliferator-Activated Receptor Gamma (PPARγ), a target of high relevance for metabolic diseases (Link to service, Link to Documentation).
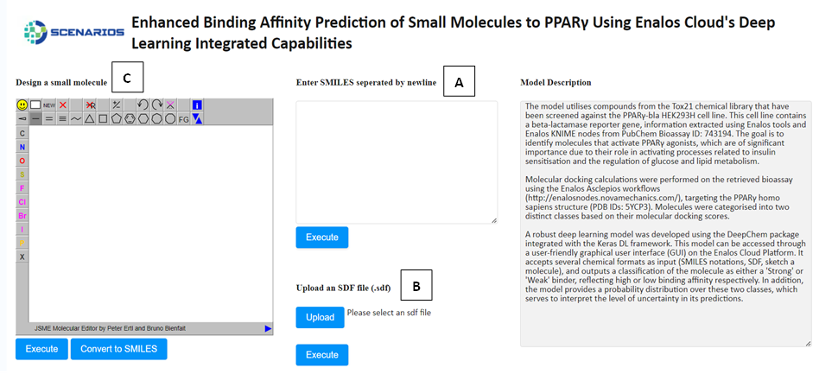
Cytotoxicity Prediction through Consensus Modeling: A synergistic approach combines deep learning predictions with molecular descriptors, enhancing the accuracy of cytotoxicity assessments of compounds. This model communicates with the previous deep learning model via Enalos APIs (Figure 3) to integrate the compounds’ binding affinity to PPARγ to the prediction of their cytotoxicity. This model employs methodologies like Random Forest, k-Nearest Neighbors, and Support Vector Machine for robust consensus predictions (Link to service, Link to Documentation).
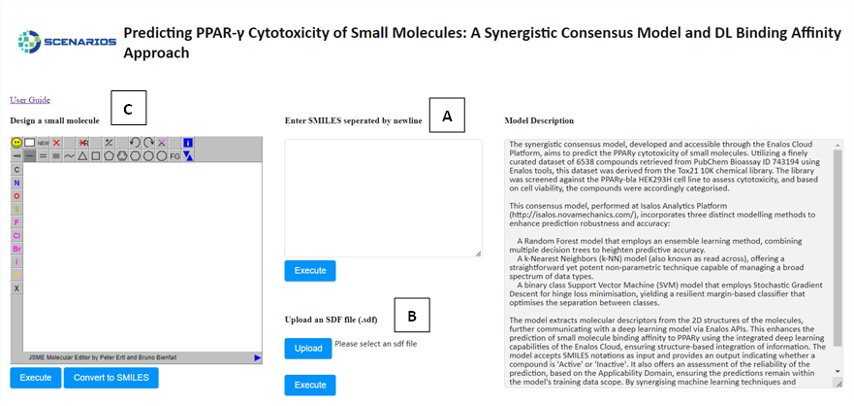
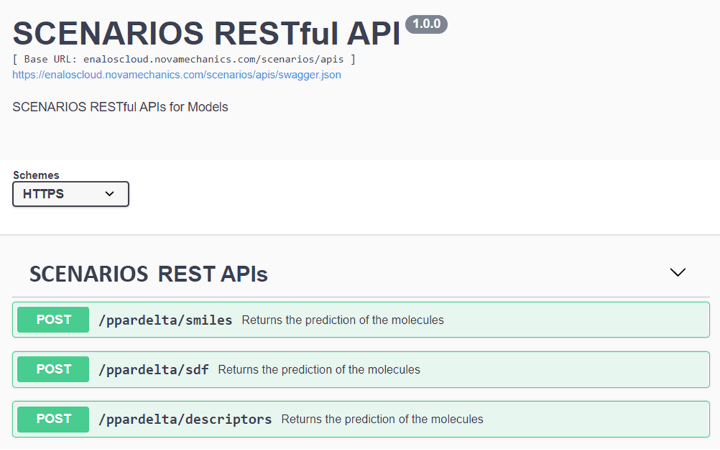
Prediction of small molecules Solubility and Bioconcentration factor: Through the SCENARIOS instance of the Enalos Cloud Platform it is possible to assess the properties and toxicity of small molecules based on two k-Nearest Neighbors models. In detail, the models can predict molecules water solubility and the bioconcentration factor (BCF). BCF is a measure of the potential of a chemical to accumulate in the tissues of living organisms (particularly aquatic organisms), potentially leading to harmful effects on them or on the ecosystem. (Link to service (logS), Link to service (logBCF))
The SCENARIOS project’s applications could significantly contribute to understanding and mitigating the environmental and health impacts of PFAS. As PFAS interact with biomolecules such as PPARα/γ and enable their transport within the body, understanding the PPAR-PFAS interactions may allow designing alternative compounds with minimal impact. In this case, by leveraging the predictive models developed for assessing small molecules’ interactions and toxicity, researchers can potentially evaluate PFAS’s effects on biological targets and their cytotoxicity. These tools offer an advanced computational approach to screen PFAS compounds efficiently, predict their environmental persistence, and assess their potential health risks, aligning with global efforts to manage and reduce PFAS exposure.
In all environments, the input environment is the same. The graphical user interface offers three tabs allowing the acceptance of either one or several structures as input in three different formats:
- The SMILES field (A), which allows the user to submit the ‘Simplified Molecular Input Line Entry System’ (SMILES) notations of several small molecules.
- The SDF field (B), which allows the user to browse and upload an .sdf file (Structure Data Files) including one or more small molecules.
- The Design Molecule field (C), which enables the user to design and submit the chemical compound of interest via a user-friendly drawing tool.
An example to showcase the use of the applications follows, using Perfluorooctanesulfonic acid, a small molecule with PubChem ID: 74483 (https://pubchem.ncbi.nlm.nih.gov/compound/74483).
- If the SMILES notation of the molecule is known, it can be directly submitted to the appropriate window (Figure 4a). In case that more than one compound needs to be assessed, their SMILES strings must be separated by a new line.
- The user can also upload the .sdf file of the compound of interest in the appropriate field (Figure 4b). The respective field accepts one .sdf file at a time.
- In the sketcher field, the user can easily draw any chemical compound and then submit it for prediction. A variety of options are available in this window for adding atoms, bonds, rings, or carbon chains anywhere in the drawing area (Figure 5).
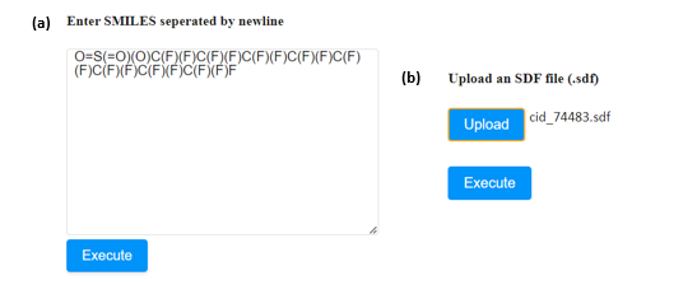
After providing the chemical structures of the molecules, the predictive model is applied to the input data and a prediction is generated within seconds by pressing the ‘Execute’ button. A table is presented that includes the respective outcome of the application in a table format. An example of Cytotoxicity Prediction through Consensus Modeling is presented in Figure 6.
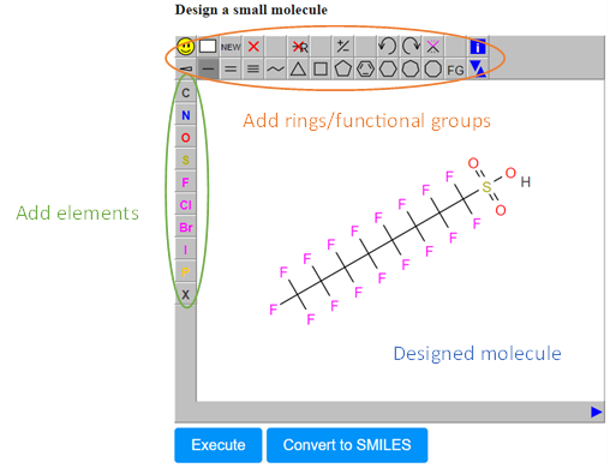
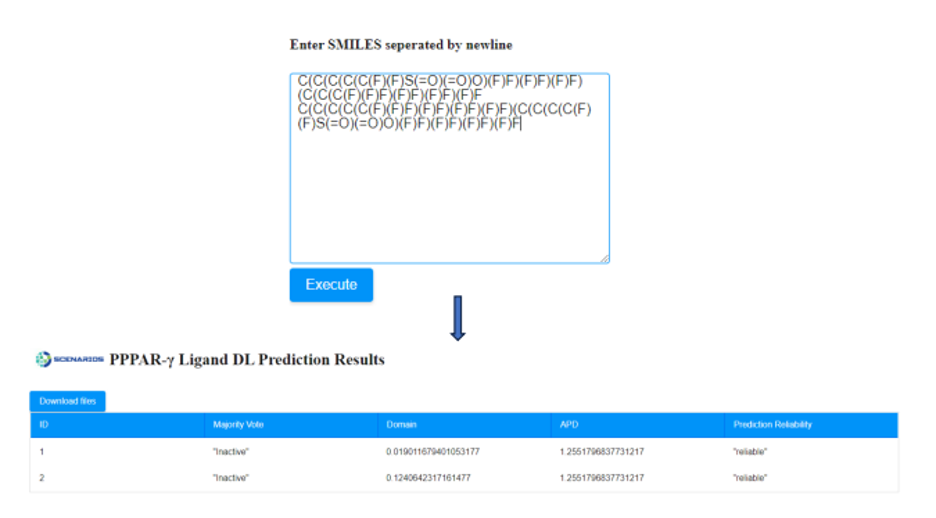
For more information, contact NovaMechanics Ltd. at: info@novamechanics.com